Researchers Develop New Diabetic Diet Monitoring Method
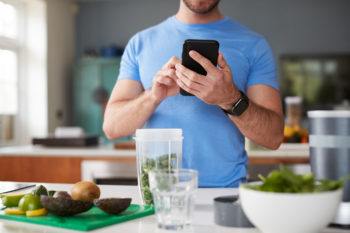
A new diet monitoring method being developed by a team of researchers at Texas A&M University could benefit the more than 30 million Americans living with Type 2 diabetes and decrease the risk of progression past prediabetes
The team at the Precise Advanced Technologies and Health Systems for Underserved Populations (PATHS-UP) center, led by principal investigator Bob Mortazavi, recently received a grant from the National Science Foundation’s Smart and Connected Health program in support of its work.
Poor diet contributes to more than half of premature deaths in the United States related to cardiovascular and metabolic disease, including Type 2 diabetes. Current methods of recording meals and tracking macronutrients — carbohydrates, protein, fat and fiber — can be inaccurate and difficult to use. Many food diaries require manual entry and require an individual to estimate how much of each macronutrient is in their food.
“Food diaries are invaluable in a number of medical interventions (e.g., diabetes, cardiovascular disease), but they are difficult to maintain since they require manual entry,” said Ricardo Gutierrez-Osuna, co-principal investigator on the study and professor in the Department of Computer Science and Engineering. “Our objective is to develop algorithms that can predict the macronutrient composition of a meal automatically by analyzing how blood glucose changes after the meal.”
Studies have shown that the shape of blood glucose changes based on intake levels of different macronutrients. The team’s solution: continuous glucose monitors with automated machine learning. If researchers can analyze the shape of blood glucose after a meal, they can reverse engineer the results to break down the macronutrient intake.
Mortazavi, assistant professor in the Department of Computer Science and Engineering, said one application of this technology would allow individuals to log the makeup of meals even when they’re in places where they have no knowledge or control over the ingredients.
“This will also enable diet intervention studies for the health care industry, understanding the changes in glucose response to meals at an individual level and allowing users to understand the differences in their responses based upon the differences in the meal macronutrient compositions, enabling clear intervention plans for changing diet and health,” Mortazavi said.
The work funded by the grant will be focused on developing the machine-learning algorithms to predict food macronutrients from glucose responses.
“We are very grateful for the opportunity to work on this project, which we view addresses a critical need in automated diet logging,” Mortazavi said. “The NSF Smart and Connected Health program has been uniquely positioned to create important new personalized sensing paradigms and analytic strategies that foster clinical care. We are excited to get started.”
The researchers are collaborating with the Sansum Diabetes Research Institute in California. The collaboration will involve data collection efforts and translation of findings toward the end of the project.
“Once the user studies and data collection are complete, the main challenge will be for us to develop the machine-learning algorithms,” Gutierrez-Osuna said. “We’re trying to solve a problem that is computationally very difficult.”
Mortazavi said a broader impact of the work is enabling patients and physicians to become better educated on how a body responds and develop a more personalized diet intervention strategy.
“This project would be helpful to clinicians to provide new information to support positive behavior change to reduce the risk of or progression from prediabetes to Type 2 diabetes, and would make it easier for patients to passively and accurately track nutritional components of their diet, potentially leading to healthier diets and improved health,” Mortazavi said.
This article by Jennifer Reiley originally appeared on the College of Engineering website.